When the weather forecast is wrong, don’t shoot the messenger
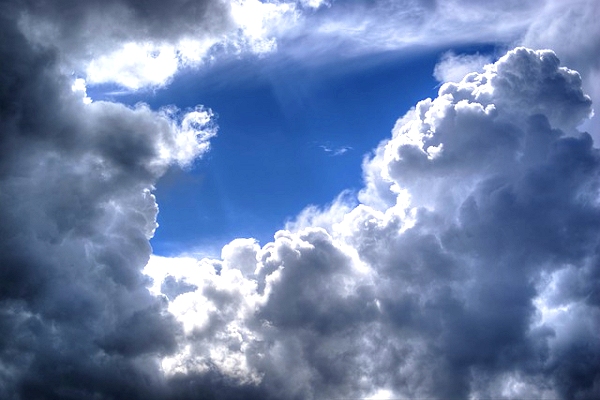
(This article first appeared last week as a guest blog on the website of the Scientific American.)
“The weather report is always wrong!” is a common refrain among people from all walks of life; it even makes headlines when high-profile politicians and football coaches blast forecasters for erroneous reports.
It’s tempting to shoot the messenger when a forecast goes awry, but if it unexpectedly rained on your picnic this summer, we suggest you take it easy on your local weatherperson.
From synthesizing temperature, pressure and wind speed data, to interpreting imperfect weather model output, there’s a lot that can go wrong.
Weather prediction is a misunderstood, frequently maligned science, so it is helpful to understand how flying balloons, complex calculus, and yes, a little bit of human judgment play into the forecast you see on TV or your new iWatch.
Meteorology is far from a guessing game. When meteorologists pore over a weather map with foreign-looking symbols, or more modernly, a computer screen with a swirling mess of colors, they’re not reading tea leaves – they’re analyzing the data products of sophisticated computer models.
Yet the result has to be simple. As News 12 Meteorologist Adam Epstein puts it, “people like to see a single number.”
We begin with what we know. Before we think about the future, we need to know what the atmosphere looks like right now.
Upper air
In fact, the majority of the skill in weather forecasting comes from the interpretation of initial conditions, the information that weather models have about what the atmosphere looks like now. Easy, right?
Anyone with a thermometer and a good sense of direction can tell you the temperature, whether or not it’s raining, and where the wind is coming from. But mere surface conditions aren’t enough to make a good forecast.
The real action – especially the kind meteorologists care about – begins up to seven miles into the sky. You can see the problem here. There is no way of knowing what is happening at every minute at every point between here and the top of the atmosphere.
Luckily, there’s a partial solution: weather balloons outfitted with an instrument package called a radiosonde are regularly launched twice a day at 92 stations in North America and the Pacific islands, a small part of the global upper air observation network.
As a balloon travels upward it records how the pressure, temperature, wind speed and direction change with altitude.
Unfortunately, 92 stations don’t come close to forming a complete picture of the atmosphere.
Take Indiana, for example. There is a 310-mile expanse between the two upper air observation stations nearest the state, which are in Wilmington, Ohio and Lincoln, Illinois. It’s an unquestionable hole in the observational system.
Before the data can be used, these holes must be filled in. Techniques vary, but the basic result is the same: filled in data can only approximate the behavior of weather variables between stations.
This is the first layer of uncertainty that meteorologists contend with. There’s another important variable when it comes to the weather: it moves.
‘Megastorms’
The same weather system over the eastern US right now may have been over the Pacific Ocean a week ago. As you can imagine, there aren’t a lot of observations available over the Pacific, so meteorologists must wait until storms reach the West Coast before they can fully trust the model output.
So when you see the media talking about a “megastorm” that may occur in a week, and a week later you get an inch of snow and wonder what the deal is, you can infer that a lack of observations played a role in the outcome.
Meteorologists love to brag about their models. But what are these models and what do they have to do with the weather?
Weather models represent another layer of the forecasting process. Models calculate how changes in wind and heat will ripple across the atmosphere to form storm systems.
The observations act as the starting point and the model predicts patterns of future weather.
But it’s not that simple. The atmosphere is dynamic and inherently chaotic. This means it is constantly moving and changing in unpredictable ways, and even small perturbations can lead to big changes in how air masses move and storms form.
Even with a better observational network and flawless models, chaos would limit how far into the future we could accurately predict weather.
Edward Lorenz described this phenomenon as “the butterfly effect” when he famously said that the flapping of a butterfly’s wing could cause a tornado half a world away.
As fantastical as that sounds, it’s theoretically true, and explains why it’s difficult to predict weather more than about a week in advance; slight changes in the initial conditions can lead to wildly different forecasts. That’s why you shouldn’t plan your vacation around Accuweather’s 25-day forecast.
To reduce this uncertainty, forecasters rely on “perturbation ensembles,” slightly changing the observations of the atmosphere before running the model.
They repeat this a number of times, until they can obtain a distribution of possible futures. So when you see a 10 per cent chance of rain, this means that 10 per cent of these individual model runs result in rain.
Human error
If this was all meteorologists were up against, it would be more than enough for them to get a pass when the forecast turns out to be wrong (or a pat on the back when it’s right). This isn’t the end of the road, however.
Forecasters utilize more than one model, and each model has its own strengths and weaknesses based on differences in how they approximate small-scale processes.
Water droplets colliding and sticking together during cloud formation are an example of a process that is too small for the model to “see” so it’s approximated instead. This approximation is not perfect, but helps to make each model unique. Looking at multiple models, forecasters balance potential biases in each one.
Meteorologists have experience using these models and can recognize situations where they perform poorly. For example, when there is snow cover on the ground, some models overestimate the temperature because they cannot “see” the snow.
A meteorologist based in a local National Weather Service office, on the other hand, is aware of the snowstorm that occurred in that forecast area over the weekend, and from experience understands that energy from the sun is partially used to melt the snow, instead of warming up the air, causing temperatures to rise more slowly.
In this way forecasters use the model output as guidance, but tweak that information based on their expertise.
When forecasters are faced with models that disagree, they must choose between them, treat each outcome as equally likely, or weigh the models based on prior experience.
This lets the forecasters adjust the model outputs to the needs of the communities they serve. However, it also introduces human error, especially when one model is chosen over another and the range of possible outcomes is inadequately communicated to the public.
Forecasters must balance these sources of uncertainty and deliver the most accurate forecast that effectively serves mayors, party-planners and joggers alike. This is a task that is anything but simple, with uncertainty at every step of the process.
And despite all the complicated effort, as News 12’s Epstein says, “a large portion of the audience simply wants to know if it will rain or not”.
(Cloudscape image: Pixabay)